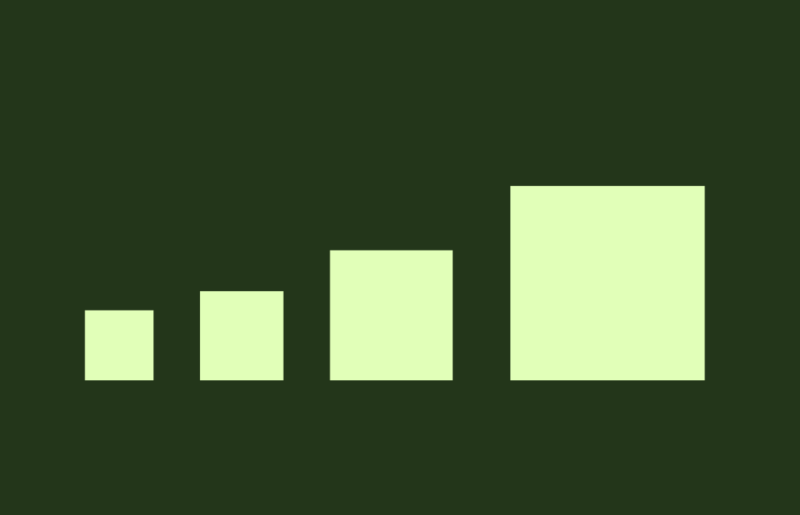
Why SMBs are prime candidates for GenAI adoption
Discover how the strategic adoption of GenAI is unlocking competitive advantages for SMBs and helping to level the playing field.
RAG represents a significant advancement in AI capabilities. Unlike traditional AI models relying solely on pre-trained knowledge, RAG combines large language models with real-time information retrieval.
The modern business landscape will reward those who can quickly access, process, and act upon information. Retrieval Augmented Generation (RAG) emerges as a game-changing technology as information processing becomes a key differentiator in the business world. This innovative approach revolutionizes how companies leverage data and artificial intelligence, offering a competitive edge in an increasingly complex marketplace.
RAG represents a significant advancement in AI capabilities. Unlike traditional AI models relying solely on pre-trained knowledge, RAG combines large language models with real-time information retrieval. This synergy produces responses that are not only intelligent but also up-to-date and contextually relevant.
The Inner Workings of RAG: A Two-Step Process
The inner workings of RAG involve a two-step process. First, when presented with a query, the system searches vast databases, including company documents, industry reports, and even the internet, to find relevant information. Then, this retrieved information is fed into a large language model, which synthesizes the data with its pre-existing knowledge to generate a tailored response. The process resembles having a tireless, lightning-fast research assistant with access to an ever-expanding library, capable of providing insights in seconds.
RAG Across Industries: Real-World Impact
RAG’s impact spans various industries. In financial services, investment firms use RAG to analyze market trends, process financial reports, and generate investment insights. A major hedge fund reported a 30% increase in trading efficiency after implementing RAG-powered analysis tools. The healthcare sector has seen RAG assist medical professionals by quickly retrieving and synthesizing information from medical journals, patient records, and clinical trials. A leading hospital network reduced diagnosis time by 40% for complex cases using RAG-enhanced decision support systems.
Law firms employ RAG to review contracts, ensure regulatory compliance, and conduct legal research. A multinational corporation saved over 5,000 billable hours in a year by using RAG for initial contract reviews. In e-commerce, online retailers enhance product recommendations and customer service with RAG. A major e-commerce platform saw a 25% increase in customer satisfaction scores after implementing RAG-powered chatbots.
Beyond Efficiency: The Broader Business Impact
The business impact of RAG extends beyond mere efficiency. Decision-makers benefit from comprehensive, up-to-date information, enabling more informed choices in rapidly changing environments. By automating complex research and analysis tasks, RAG frees up valuable human resources for higher-level strategic work. The technology allows businesses to provide personalized experiences to customers and tailored insights to employees, all while operating on a global scale. Moreover, by ensuring access to the latest regulatory information and market trends, RAG helps businesses stay compliant and ahead of potential risks.
Challenges in RAG Implementation
Retrieval Augmented Generation (RAG), while powerful, has several limitations. The quality and relevance of RAG’s outputs heavily depend on the accuracy and comprehensiveness of the knowledge base it retrieves from, potentially leading to outdated or biased information if the source data is flawed. RAG systems can be computationally intensive and may face challenges with real-time performance, especially when dealing with large-scale data. They also struggle with understanding complex contexts or nuanced queries that require deep reasoning beyond simple information retrieval. Privacy and security concerns arise when handling sensitive or proprietary information in the retrieval process. Additionally, RAG may sometimes retrieve irrelevant information, leading to unnecessary noise in the generated responses. The technology also faces challenges in maintaining coherence across long-form content generation and can be limited by the fixed nature of its retrieval database, which may not capture very recent information unless constantly updated. Finally, RAG systems may still produce hallucinations or incorrect information, especially when faced with ambiguous queries or gaps in the knowledge base.
Data orchestration emerges as a crucial solution to overcome the limitations of RAG systems. By efficiently managing, integrating, and synchronizing data from diverse sources, data orchestration ensures that RAG systems have access to high-quality, up-to-date, and relevant information. This approach not only enhances the accuracy and reliability of RAG outputs but also addresses challenges related to data quality, real-time performance, and the handling of complex, multi-modal information, thereby significantly improving the overall effectiveness and applicability of RAG in various business contexts.
Strategic Approach to RAG Implementation
For businesses looking to implement RAG, a strategic approach is crucial. Starting with an assessment of the current data infrastructure helps identify areas where RAG could have the most significant impact. Piloting RAG in a specific department or for a particular use case before a company-wide rollout can provide valuable insights. Investing in training ensures teams are well-versed in both the capabilities and limitations of RAG technology.
Do you want a single AI platform that works for your business?
For two years, Ragu has learned how to deploy foundational gen AI models into companies at scale. Ragu is now a platform. Ragu empowers businesses of all sizes to easily create custom AI assistants with advanced security and cutting-edge technology. Ragu’s RAG system provides a comprehensive and reliable solution that goes beyond the capabilities of standard RAG implementations The Ragu AI system demonstrates a sophisticated approach to handling user queries. By combining custom search capabilities, knowledge bases, and large language models, it provides responses that are not only accurate but also contextually rich and informative.
The Ragu AI system is a sophisticated digital assistant platform that combines custom search capabilities with large language models to provide enhanced responses to user queries.
The Ragu AI Workflow
The Future of RAG: Emerging Trends
As RAG technology evolves, several exciting trends are emerging. Future systems will likely incorporate not just text, but also images, audio, and video in the retrieval and generation process. Advancements in federated learning allow RAG systems to leverage distributed data sources while maintaining privacy. RAG systems may soon be able to learn and adapt in real-time, continuously improving their performance based on user interactions
RAG as the Future of Business Intelligence
LLMs excel at processing unstructured data, effectively unlocking the knowledge contained in documents and presentations. This capability allows us to incorporate this valuable information into the context we provide, enhancing the overall relevance and accuracy of the AI’s responses. Read more about this here.
Retrieval Augmented Generation stands at the forefront of the AI revolution in business intelligence. By bridging the gap between vast knowledge bases and specific business contexts, RAG offers unprecedented opportunities for efficiency, innovation, and competitive advantage. As technology continues to evolve, it promises to unlock new possibilities in business intelligence, limited only by imagination and willingness to embrace change.
The future of business intelligence lies in the ability to access information in a way that is context-aware, up-to-date, and directly applicable to the task at hand. RAG represents a significant step towards this future, offering businesses a powerful tool to navigate the complexities of the information age. Those who thoughtfully embrace this technology will be best positioned to thrive in an increasingly data-driven world.